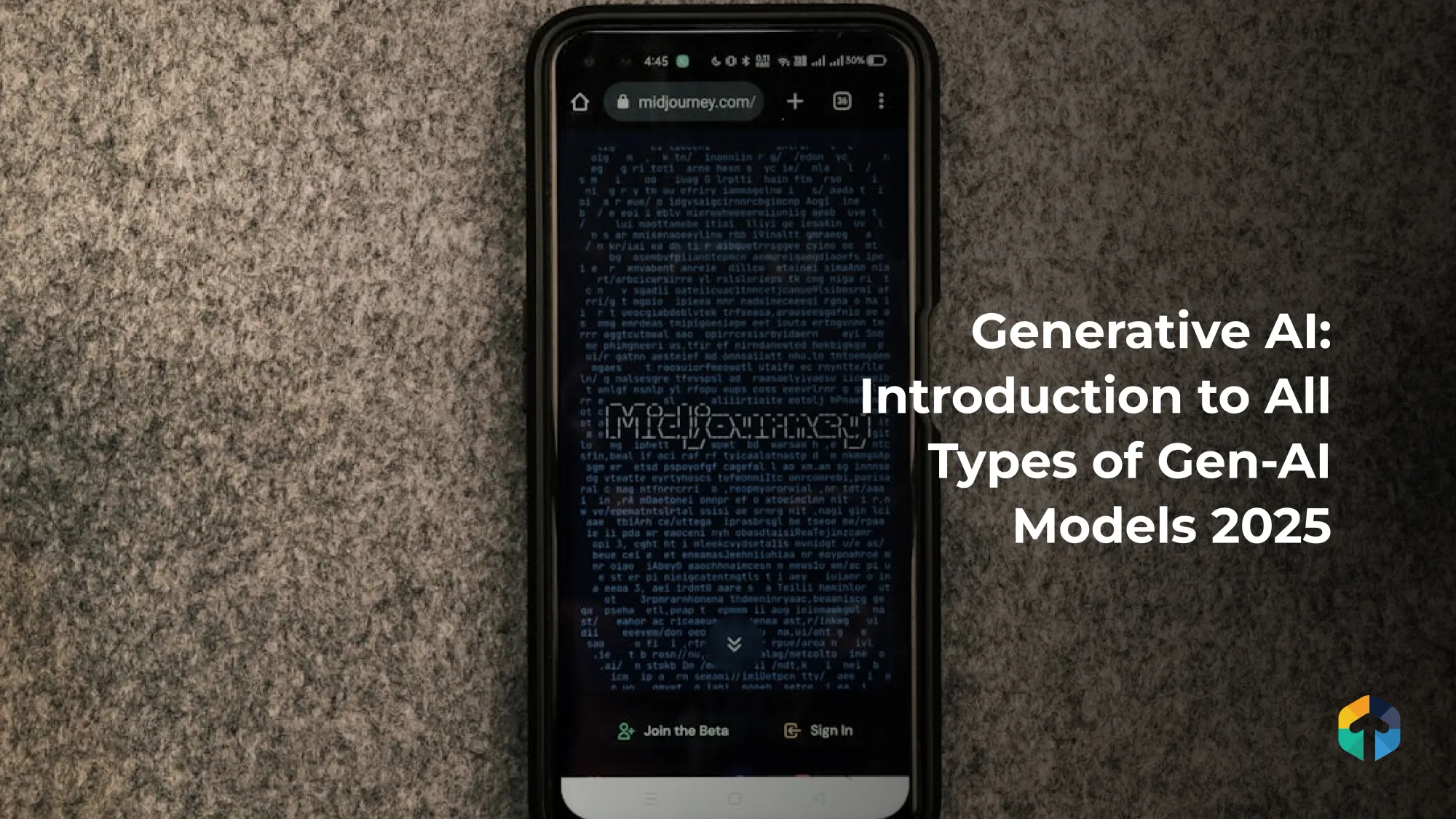
AI offers capabilities that extend beyond what we once thought possible. Today, Gen-AI is creating life-like images and writing human-like text. Generative AI models are becoming indispensable across various industries. This blog will explore the different types of generative AI models, how they work, and their multiple applications.
Table of Contents
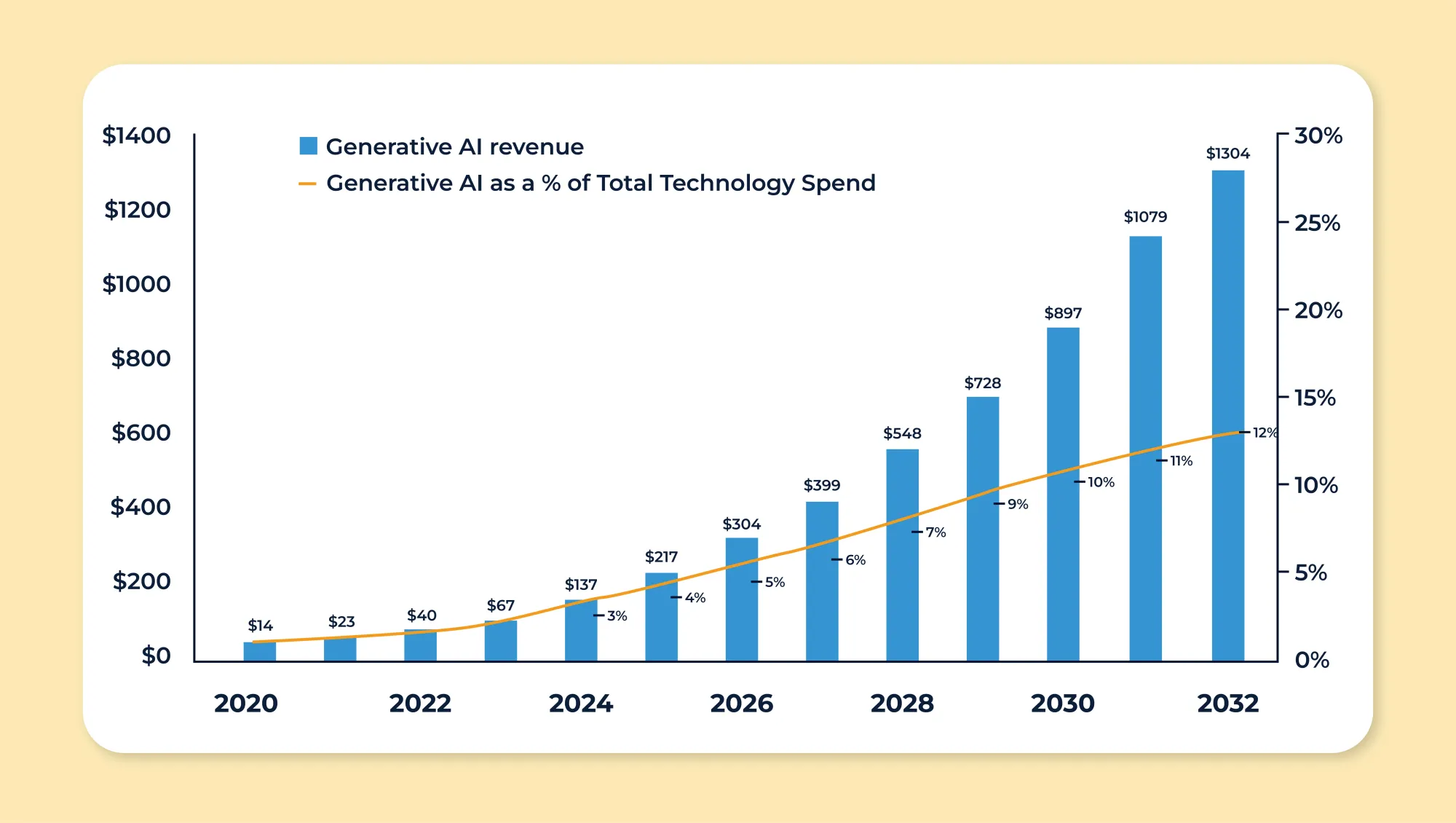
Let’s jump into the core elements of Generative AI, its use cases, and potential challenges.
What is Generative AI?
Generative AI relies on much more sophisticated machine learning models called deep learning models. This deep learning models algorithms that simulate the learning and decision-making processes of the human brain. These models work by identifying and encoding the patterns with relationships in huge amounts of data.
We all know AI has been one of the hot technologies and topics for the past few years, but I think generative AI arrive at ChatGPT in 2022. Generative AI offers many productivity benefits for individuals as well as organizations. Seriously, while there are many challenges and risks, businesses are still moving ahead and exploring how this technology can improve their internal workflow.
According to research by the management consulting firm McKinsey, one-third of organizations are already using generative AI regularly. Another industry analyst, Gartner, states over 80% of organizations will deploy generative AI applications or programming interfaces.
How Do Generative AI Models Work?
At the heart of Generative AI are complex algorithms designed to mimic human creative processes and solve complex problems as well. Here's a step-by-step breakdown of how these models function.
For the most part, generative AI operates in three phases
Training to create a foundation model that an serve as the basis of multiple-gen AI applications.
Tunning to customize, made the function model for a specific gen AI application.
Generation, evaluation, and return to assess the gen AI application's output and continually improve its performance and accuracy.
Training Gen AI
Generative AI begins with a foundation model- a deep learning model that serves as the basis for multiple different generative AI applications. The most common foundation model today is LLMsm, which was created for text generation applications. There are also foundation models for image generation, video generation, and sound and music generation. On top of that, multimodal foundation models can also support several kinds of content generation. (Was it too much, hey?)
Moving on, the result of this training is a neural network of parameters-encoded representations of the entities, patterns, and relationships in the data- that can generate content autonomously in response to inputs or prompts.
Tuning the Gen AI
Tunning involves feeding the model labeled specific data to a content generation application, like questions or prompts the application is likely going to receive from the users. For example, if a development team is trying to create a customer services chatbot, it would create hundreds or thousands of documents containing labeled customer services questions and correct answers and then feed those documents to the models.
Fine-tunning is labor-intensive. Developers often outsource the task to companies with large data-labeling workforces.
Fine-tunning is labor-intensive. Developers often outsource the task to companies with large data-labeling workforces.
Reinforcement learning with human feedback
In RLHF, human users respond to generated content with the model, which can be used to update the model for better accuracy and relevancy. Often, RLHF involves people scoring different outputs in response to the same prompt.
Generation, Evaluation, and Fine Tunning
This is the stage where developers and users continually assess the output of their generative AI apps and then tune them if needed. Another option for improving a Gen AI model is retrieval augmented generated (RAG). RAG is a framework for extending the foundation of the generative model. Using relevant sources outside of the training data and supplementing them also refines the parameters or representations in the original mode. RAG can make sure that a generative AI app always has access to the most current information.
The Core Types of Generative AI Models
There are several types of models, each with its unique characteristics and applications. Let's break down the most used one.
Generative Adversarial Networks (GANs): Evolution and Application
Generative Adversarial Networks (GANs) consist of two neural networks, the generator and the discriminator, that work in tandem. The generator creates new data while the descriminator evaluates its authenticity. This process helps GANs improve over time, producing high-quality results. Task-Specific GANs: Image and Video ImprovementTask-Specific GANs are particularly useful in improving the quality of Images and videos, from photo restoration to video upscaling. GAN helps create sharper and more appealing content. Security and Privacy Use Cases of GANsOk, In addition to media improvement, GANs have security applications. They can create synthetic data for training models, allowing organizations to safeguard sensitive information by using data that can mimic the real thing.Transformed-Based Model: Driving Modern AI
Transformer-based models have brought generative AI into the mainstream. These models understand context, making them ideal for tasks like language processing and content generation. GPT Series: From GPT-4 to LLaMa by MetaThe GPT series (Generative Pre-Trained Transformer) represents some of the most powerful based models. While GPT-4 has garnered attention for its human-like text generation, Meta’s LLaMA has also contributed significantly to the development of more efficient models.BERT: Powering Naturel Language Processing (NLP)BERT (Bidirectional Encoder Representations from Transformers) is another transformer model that excels in NLP tasks, helping AI understand context and meaning in ways that traditional models cannot.Diffusion Models: The Mechanism Behind Image Creation
Diffusion models are a newer type of generative AI that excels in tasks like image generation by simulating the process of image formation.Stable Diffusion: A Deeper LookStable Diffusion has become very popular for its ability to create detailed and accurate images. It generates images by gradually removing noise from an initial random image, producing a clear and cohesive result.DALLE: Image GenerationWe all know Open AI’s DALLE model takes image generation a step further, allowing for text-to-image transformations. It can generate unique visuals based on text input, making it a revolutionary tool in creative fields.Variational Autoencoders (VAE): Flexible Generative AI Models
Variational Autoencoders (VAE) are widely used in generating diverse types of content, from images to audio files.VAEs in Image and Audio GenerationVAEs are highly effective in creating realistic images and, to some extent, musical ideas. Their ability to to compress data into smaller representations and expand it allows for efficient generation across various media types.Security & Privacy Application of VAEsMuch like GANs, VAEs can be used to generate synthetic datasets, providing a secure alternative for data handling in sensitive fields like healthcare and finance.Hybrid Models In Generative AI
One of the latest advancements in the field of generative AI is the development of hybrid models, which combine various techniques to create innovative content generation systems. These models draw on the strengths of different approaches, like GAN with diffusion models to refined and realistic output. On the other hand, LLMs can also be integrated with other neural networks, and these hybrid models can offer improved content and adaptability. You might have guessed it: this hybrid model unlocks new possibilities for applications. I think DeepMind’s AlphaCode is a great example. It combines LLMS and adaptive learning to generate high-quality commuted code.
Let's start building your project
Get a free consultation to transfrom your productideas into
reality
What kind of Content can Generative AI Create?
Now, you know everything about Gen AI and how it works. It is time for a real question. What are the things Generative AI can accurately generate?
Generative AI can create many types of content across many different domains.
Text
You show it coming, of course, in text. Generative models, especially those based on transformers, can generate coherent, contextually relevant text- everything from instruction to documentation. Brochures to email, website copy to blogs, and much more. They can also perform repetitive tasks. This is good to some extent and helps writers focus on creative and highly valued work.Images and Video
We all know models like DALL-E and MidJourney are known for their image and video creation. This model uses stable diffusion and can generate realistic images, image-to-image translation, and other image editing and enhancement tasks. These tools can also create text-to-video with special effects, and I think this is quicker and more cost-effective than other methods.Sound, Speech, and Music
Generative models can also synthesize natural-sounding speech and audio content for voice-enabled AI chatbots and digital assistants. The same tech is also can generate original music that mimics a professional composition.Simulations and Synthetic Data
Generative AI models can be trained to generate synthetic data or synthetic structures based on real or synthetic data. For example, generative AI can help in drug discovery to generate molecule structures with its properties.Design and Art
Generative AI models can generate works of art and design or assist in graphic design. Applications include dynamic generation of environments, characters, and even avatars with special efficiency in video games.
Benefits of Generative AI in Various Fields
I think what we can get from AI is efficiency. Because it can automate repetitive tasks, decrease labor-intensive tasks, cut costs, and save time. Let's look at some of the benefits for individuals and organizations.
Improved Creativity
Gen AI tools can inspire creativity through automated brainstorming, which generates multiple novel versions of content. These variations can also serve as good starting points or references that help writers, artists, and other creators plow through creative blocks.Improve Decision-Making
Generative AI excels at analyzing large datasets, identifying patterns, and extracting meaningful insights. It can also generate hypotheses based on those insights to support executives and analysts.Constant Availability
Generative AI can work 24/7, continuously without any stress, providing support around the clock, helping customers, and automating processes.Dynamic Personalization
There are very few real-world examples, but I think this can improve user experience by a good margin. Gen AI can analyze user preferences and history and start generating personalized content in rea-time.
Real-World Application of Generative AI
The following are some of the handful of use cases of gen AI for enterprise. As technology develops and organizations integrate tools into their workflows, we can expect to see many more.
Improve Customer Experience
Marketing organizations can save time and amp up their content production. It can generate content like blogs, web pages, collateral, email, and more. AI solutions can also produce highly personalized marketing copy and images as well. On the other hand, chat and virtual agents can give personalized responses and even initiate actions on behalf of customers.Software Coding Generation
We all know that code generation tools can automate and increase the process of writing new code. Code generation also has the potential to accelerate application modernization by automating much of the repetitive coding required to modernize applications.Digital Labor Work
Generative AI can quickly draw contracts, invoices, bills, and other digital 'paperwork' so that employees who use or manage it can focus on higher-level tasks.Science, engineering, and research
Generative AI models can help scientists and engineers propose novel solutions to complex problems. Especially in healthcare, for example, gen AI can applied to synthesize medical images for training and testing medical imaging systems.
Top 5 Security and Compliance Issues in Generative AI
Deepfakes:- The potential for AI-generated fake media has raised concerns about misinformation and privacy violations.
Copyright Infringement:- Generative AI often uses pre-existing content for training, which could lead to unauthorized reproduction.
Data Security:- Sensitive data used for training models can sometimes be reverse-engineered, compromising privacy.
Bias and Fairness:- Generative AI models may carry biases inherent in the training data, leading to discriminatory outputs.
Accountability:- Determining responsibility for AI-generated content is a legal and ethical gray area.
Talk to our experts!
Get a free consultation to transfrom your productideas into
reality
Conclusion
Thats it! Generative AI is, for sure, a groundbreaking technology that is changing rapidly. With its ability to lifelike content and solve complex problems, its applications are limitless. On the other hand, there are a few challenges that need to be addressed by understanding the different types of generative AI models and their use cases.
If you are looking for a Gen AI development company, we at Third Rock Techkno have a decade of experience in custom software development, and we can help you integrate AI or develop a completely new platform custom-made for your business. Contact us now!
FAQs
1. What is Generative AI?
Generative AI is a type of AI that can create new content, such as images, text, or audio, from existing data.
2. How do GANs work?
Generative Adversarial Networks (GANs) consist of two neural network generators and the discriminator working together to create realistic outputs by constantly improving through feedback.
3. What are transformer-based models used for?
Transformer-based models are commonly used in language tasks, such as text generation and translation, due to their ability to understand content better than other AI models.
4. What are diffusion models?
The diffusion model generates images by simulating the process of noise reduction, making them ideal for tasks like image generation.
5. What are the ethical concerns surrounding generative AI?
Ethical concerns include issues like deepfakes, data privacy, copyright infringement, and the potential bias present in AI-generated content.